What is Predictive Analytics?
Predictive analytics uses machine learning to generate predictive models that can predict future events and trends.
Predictive analytics use enormous amounts of historical data to build mathematical models. These models can be used to predict future events and trends. Machine learning is an essential tool in developing predictive analytics.
The typical output of predictive analytics is a predictive model that captures the relationships that the machine learning process has discovered within the data. This model can then be used to predict the type and likelihood of future events. Typically, historical data is used to build a mathematical model that captures important trends. For example, by analyzing sales and weather data, it might determine that a retailer’s ice cream sales increase 15% when the temperature exceeds 75F.
One Network's Perspective on Predictive Analytics
The value of predictive analytics to supply chains is obvious. It helps organization anticipate events, sales, orders, and even highlight possible problems in their supply chains. This helps them better serve customers, reduce the costs and the risk of supply chain disruption.
However, machine learning requires large volumes of data in order to accurately identify relationships and trends, and few enterprises have sufficient data to make effective use of machine learning in their supply chains.
One Network’s Real Time Network consists of tens of thousands of companies managing their logistics and supply chains. NEO, One Network’s intelligent agent technology, thus has much more data than the typical enterprise, which leads to better
Furthermore, predictive analytics require action based on predictions, so by itself it is of limited use, and really needs to include prescriptive analytics, and be incorporated into a platform that spans both planning and execution, so that plans can be adjusted and execution can be optimized based on predictions.
Predictive Analytics Resources
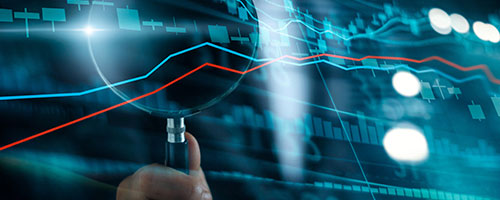
AI and Supply Chain Problem Solving
What does it take to effectively address challenges in the supply chain? What’s really required is real-time “always on” planning and execution capabilities that eliminate the information lead times between unplanned shifts in consumer demand or supply capability.
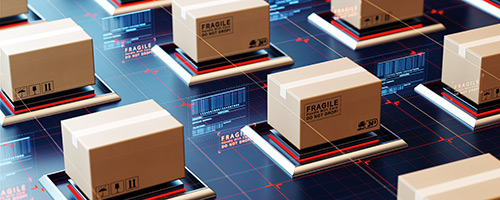
Webinar: 8 Keys to Achieving Success with AI in the Supply Chain
The success of AI in supply chains is heavily dependent on information accuracy, data timeliness, and selection of the correct decision space for AI applications. In this webinar, Joe Bellini explains the basics of AI that supply chain pros need to know, and how they can get the most from their AI implementations.
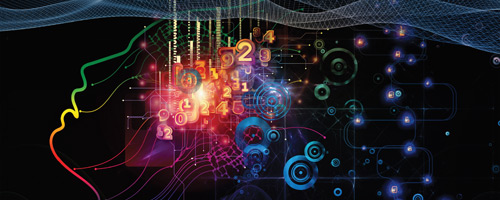
8 Keys to Success with Artificial Intelligence in the Supply Chain
Artificial Intelligence (AI) can offer a huge benefit to supply chain managers, but only if it is based on solid fundamentals that take into account the diverse and dynamic nature of today’s modern supply chains. This report identifies the eight fundamentals that need to be in place in order to achieve the dramatic returns that AI is capable of delivering.